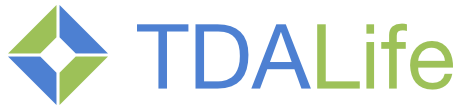
CoVtRec
Topological surveillance of potentially adaptive mutations in the evolution of
the coronavirus SARS‑CoV‑2
Michael Bleher,
Lukas Hahn,
Maximilian Neumann,
Andreas Ott
Karlsruhe Institute of Technology and Heidelberg University, Germany
Reports
- CoVtRec report as of 2024-01-10
- CoVtRec report as of 2023-11-25
- CoVtRec report as of 2023-10-31
- CoVtRec report as of 2023-09-22
- CoVtRec report as of 2023-08-22
- CoVtRec report as of 2023-07-31
- CoVtRec report as of 2023-06-28
- CoVtRec report as of 2023-06-02
- CoVtRec report as of 2023-04-26
- CoVtRec report as of 2023-03-24
- CoVtRec report as of 2023-02-27
- CoVtRec report as of 2023-01-29
- CoVtRec report as of 2022-12-25
- CoVtRec report as of 2022-10-21
- CoVtRec report as of 2022-08-22
- CoVtRec report as of 2022-07-21
- CoVtRec report as of 2022-06-17
- CoVtRec report as of 2022-05-17
- CoVtRec report as of 2022-04-28
- CoVtRec report as of 2022-03-15
About
CoVtRec is a custom Python pipeline for the computation of the topological recurrence index (tRI) for mutations in the evolution of the genome of the coronavirus SARS‑CoV‑2. The topological recurrence index is a quantifier for the potential adaptiveness of mutations. Our approach relies on Topological Data Analysis (TDA), a mathematical approach to data analysis that extracts features of high-dimensional complex data sets by analyzing their geometric shape. We use persistent homology to compute the topological recurrence index. CoVtRec systematically detects convergent events in viral evolution merely by their topological footprint in the phylogeny, overcoming limitations of current phylogenetic inference techniques. Due to highly optimized algorithms it easily scales to hundreds of thousands of distinct genomes. We provide regular reports about potentially adpative mutations based on current SARS‑CoV‑2 genome data from GISAID.
CoVtRec uses hammingdist for the computation of genetic distance matrices and Ripser for the computation of persistent homology. It implements a new approach based on the MuRiT algorithm for Vietoris-Rips transformations in multiparameter persistent homology. This leverages the stratification by time of genomic data for efficient tRI time series analyses on a daily basis.
If you have any comments or suggestions, or would like to use the CoVtRec pipeline in your own work, feel free to contact us.
Cite this work
- Topological data analysis identifies emerging adaptive mutations in SARS‑CoV‑2
M. Bleher, L. Hahn, M. Neumann, J. Á. Patiño-Galindo, M. Carrière, U. Bauer, R. Rabadán, A. Ott
arXiv:2106.07292 - MuRiT: Efficient computation of pathwise persistence barcodes in multi-filtered flag complexes via Vietoris-Rips transformations
M. Neumann, M. Bleher, L. Hahn, S. Braun, H. Obermaier, M. Soysal, R. Caspart, A. Ott
arXiv:2207.03394
Acknowledgements
We acknowledge the use of de.NBI Cloud within the German Network for Bioinformatics Infrastructure (de.NBI) and the support by the High Performance and Cloud Computing Group at the Zentrum für Datenverarbeitung of the University of Tübingen and the German Federal Ministry of Education and Research (BMBF) through grant no 031 A535A. We acknowledge support from Steinbuch Centre for Computing (SCC) at Karlsruhe Institute of Technology.